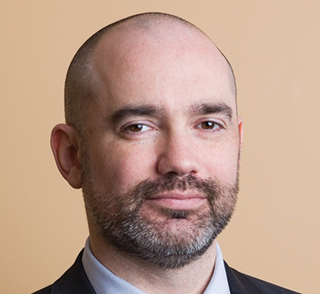
CIM Distinguished Lecturers
Guy Desharnais obtained his PhD from the University of Manitoba, which focused on belt-scale geochemistry of mafic-ultramafic rocks and related sulphide mineralization. He worked for five years as an exploration geologist with Xstrata Nickel (Glencore). Since joining SGS in 2010, Desharnais has completed a wide range of projects internationally, from resource estimations, economic evaluations, metallurgical sample selection, geometallurgical studies and audits of resources and reserves.
Desharnais has prepared NI 43-101 technical reports on a wide range of commodities and is considered a Qualified Person for a number of deposits types. He has a passion for sharing his technical knowledge and has completed several training sessions for a variety of audiences in French, English and Spanish. He led the team which won the Integra Gold Rush Challenge in 2016 ($500k) which applied a combination of geology, virtual reality, weight of evidence and machine learning techniques to identify the most prospective exploration targets.
Distinguished Lecturer 2017-18
Lecture Abstracts
Metallurgical Sample Selection: A Simple Step that has the Potential to Sink your Project
Many metallurgical programs commonly suffer from samples that are not representative of the “rock recipes” that will eventually see the inside of a processing facility. Unfortunately, this often introduces a bias that is transmitted through all the detailed technical studies all the way to the construction of a flawed processing plant. The most common blunders include selection that is limited to the richest ore type, to a single host rock, to rocks excluded from the mine plan, or the lack of consideration for diluting rock types.
Discussion will focus on the appropriate scale of characterization including the introduction of the concept of time, mine schedules and plant scale into the selection process. The lack of consideration given to appropriate sample selection directly impacts the forecasts for hardness/recovery, flowsheet design and ultimately profitability of mining projects.
Mining BIG Data: the Future of Exploration Targeting
The application Machine Learning enhances our capability to harness exploration data to establish vectors to ore. However, careful consideration of the inputs and outputs by human geologists is required to ensure that the model does not merely predict what is already known or produce spurious results.
Resource Over-Estimation: Post-Mortem and a Path Forward
Several high-profile mineral exploration projects have recently suffered major setbacks following significant reductions in Resource and Reserve estimates. This talk explores the root causes for these, as well as some possible solutions.